Fractal Classification Method for Complex Signals
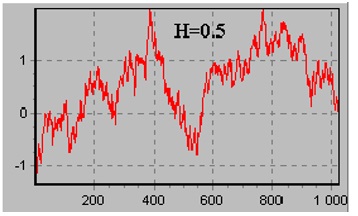
The classification of complex signals, often characterized by high noise levels, non-linearity, and overlapping patterns, poses significant challenges in signal processing. Traditional methods frequently fail to address the intricate structures inherent in such signals, necessitating the adoption of advanced analytical techniques. This study explores the application of fractal methodologies, leveraging their self-similar and scale-invariant properties, for classifying complex signals. By employing tools such as the Hurst exponent and the (R/R) method, this work demonstrates how fractal analysis can effectively characterize and categorize stationary and non-stationary signals based on their probabilistic distributions and fractal dimensions. Results indicate that fractal methods provide robust descriptors for distinguishing signal types, enabling enhanced accuracy and operational efficiency. The proposed approach holds promise for developing virtual analyzers with expansive dynamic ranges, applicable in diverse fields such as diagnostics, control systems, and signal processing.
Downloads
Metrics
No metrics found.
Eke, A., Hermann, P., Kocsis, L., & Kozak, L. R. (2002). Fractal characterization of complexity in temporal physiological signals. Physiological Measurement, 23(1), R1-R38.
Otkhozoria, N., Azmaiparashvili, Z., Petriashvili, L., Otkhozoria, V., & Akhlouri, E. (2023). Labview In The Research Of Fractal Properties Of The Topology Of Networks And Stochastic Processes. World Science. doi:https://doi.org/10.31435/rsglobal_ws/30092023/8020
Otkhozoria, N., Otkhozoria, V., Narchemashvili, M. (2021) Fractality Of Measurements Of Quantities And Real Processes. International Trends In Science And Technology, Engineering Sciences June 2021 Doi: https://doi.org/10.31435/rsglobal_conf/30062021/7620
Falconer, K. (2013). Fractals: A Very Short Introduction. Published by Oxford University Press, 138-152 page.
Abelashvili, N., Otkhozoria, N., Otkhozoria, V., & Akhlouri, E. (2024). Diagnosing the stability of large-scale processes using fractal structure analysis of time series. International Science Journal of Engineering & Agriculture, 3(4), 30–37. https://doi.org/10.46299/j.isjea.20240304.03
Copyright (c) 2025 Georgian Scientists

This work is licensed under a Creative Commons Attribution-NonCommercial-NoDerivatives 4.0 International License.